APPLICATIONS FOR AI IN HEALTHCARE & LIFESCIENCES - Part 2
- Harit Shukla
- Sep 16, 2021
- 3 min read
Part of the blog post covering uses cases of application of AI in Healthcare and life sciences.
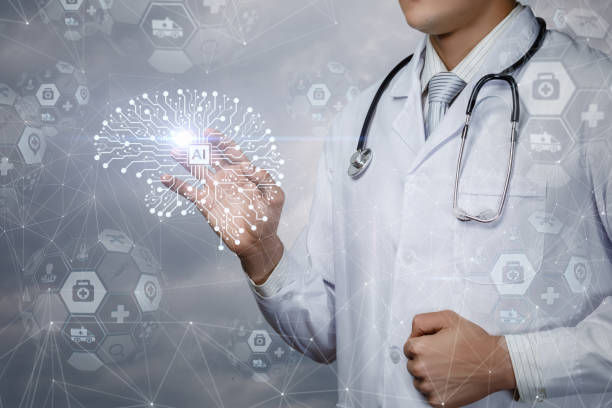
Welcome to the part 2 of the blog post covering some useful applications of AI and related tech mainly in life sciences domain.
“Do you have a design in mind for your blog? Whether you prefer a trendy postcard look or you’re going for a more editorial style blog - there’s a stunning layout for everyone.”
You’ll be posting loads of engaging content, so be sure to keep your blog organised with Categories that also allow visitors to explore more of what interests them.
PHARMACOVIGILANCE

Millions of adverse events are reported each year, and the volume is growing by about 10%- to 15% annually, compounded by data generated from new sources, such as wearables and social media. Processing these adverse event reports is a manual, costly, and often decentralised process that can impact compliance with safety regulations and prevent early discovery. But automating and centralising the intake of adverse event reports from various sources—leveraging AI-enabled technologies such as optical character recognition and natural language processing—can reduce case documentation workload to significantly expedite the investigation and reporting process.
Predicting an adverse event/effect for a drug is also an area of high interest and has a very high impact on patients.
CLINICAL TRIALS
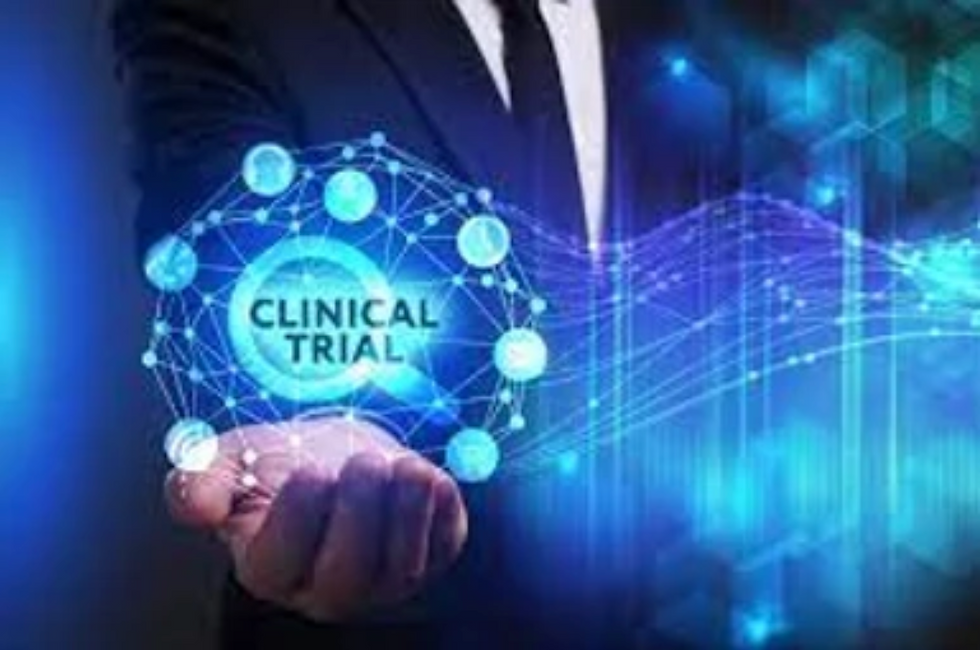
AI is helping clinical trial data managers uncover insights from clinical trials that can lead to safer, more efficient trials.
“When used to automate clinical trials, AI can significantly reduce cycle times and costs, while also improving the outcomes of clinical development.”
Machine learning has several potential applications which may help direct clinical trial research. For example, applying advanced predictive analytics in identifying candidates for clinical trials could provide a much wider range of data, including social media and doctor visits, as well as genetic information on the target populations. Better sampling techniques would lead to smaller, quicker, and less expensive trials.
ML can also be used for remote monitoring and real-time data access; for example, constant monitoring of biological signals for any sign of harm to participants or their eventual death. Some other possibilities for ML applications include finding the best sample sizes; addressing and adapting to differences in sites for patient recruitment; and using electronic medical records to reduce data errors.
DRUG DISCOVERY

AI is transforming R&D by applying data science and machine learning to massive data sets, enabling rapid discovery of new molecules. AI in drug discovery can cross-reference published scientific literature with alternative information sources, including clinical trial information, conference abstracts, public databases, and unpublished data, to surface promising therapies.
Machine learning is a powerful tool in preliminary (early-stage) drug discovery which may be used in a range of activities, from initial screening of drug compounds to success rate prediction based on biological factors and to R&D discovery technologies like
Drug discovery is often one of the longest and most costly parts of drug development. AI could help reduce the costs of developing new medicines in primarily two ways: creating better drug designs and finding promising new drug combinations. With AI, many of the big data challenges facing the life sciences industry could be overcome.
DECISION MAKING

Improving care requires the alignment of big health data with appropriate and timely decisions, and predictive analytics can support clinical decision-making and actions as well as prioritise administrative tasks.
Using pattern recognition to identify patients at risk of developing a condition – or seeing it deteriorate due to lifestyle, environmental, genomic, or other factors – is another area where AI is beginning to take hold in healthcare.
DRUG RESEARCH AND DISCOVERY
The path from research lab to patient is a long and costly one. According to the California Biomedical Research Association, it takes an average of 12 years for a drug to travel from the research lab to the patient. Only five in 5,000 of the drugs that begin preclinical testing ever make it to human testing and just one of these five is ever approved for human usage. Furthermore, on average, it will cost a company US $359 million to develop a new drug from the research lab to the patient.
Drug research and discovery is one of the more recent applications for AI in healthcare. By directing the latest advances in AI to streamline the drug discovery and drug repurposing processes there is the potential to significantly cut both the time to market for new drugs and their costs.
Current challenges in Drug discovery also has potential solutions using the next frontiers in tech like Quantum Computing
Comentários