APPLICATIONS FOR AI IN HEALTHCARE & LIFESCIENCES - Part 1
- Harit Shukla
- Sep 17, 2021
- 8 min read
Interesting use cases of AI and ML in Healthcare and Life sciences. Part 1 of this post enlists some of them in Healthcare.
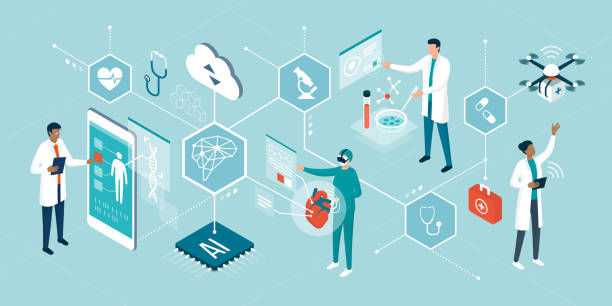
AI is everywhere and is being infused in our life in so many ways. For those who are in this field or are following it would be curious and aware how it is being used, as an example in an application like Google Maps and for others, it's how the application "just works".
I work with AI/ML engineers to design and build products and hence I continuously self-learn and learn on the job in the field of AI and have been fortunate to work in healthcare(pharma) which is one of the high impact areas where these technologies are used.
As I read about the applications of AI in healthcare in various research papers, regulatory websites , some social media sites and experience some of them in the work I do, I wanted to capture some of my thoughts around them and repeat some which I have read about which are practical use cases. These are just a few of them from a vast array of applications out there.

The following are some of the exciting use cases in Healthcare.
PATIENT - PHYSICIAN EXPERIENCE
This is a big area and opportunity for AI to improve the end to end patient experience. Though different companies work in specific use cases and hospitals and medical facilities use them accordingly, I believe the future will have a more comprehensive suite of AI tools behind a more seamless and efficient patient experience from their homes to the hospital and back and further for future follow-ups.
“I believe the future will have a more comprehensive suite of AI tools behind a more seamless and efficient patient experience from their homes to the hospital and back and further for future follow-ups”
Conversational AI has practical use cases in improving how patient care is given and improving the quality of the overall experience.
Few examples:
Nuance-DAX (Nuance - Conversational AI for Healthcare and Customer Engagement | Nuance) which offers Unified, Omni-channel, integrated digital patient engagement experience
Suki (Suki - AI Assistant | Medical Dictation Software which delivers voice experiences using NLP)
There has been a growing need for accurate and organised information where AI can play a vital role.
An example : Care Studio: Clinical Software - Google Health
Chatbots
The number of healthcare chatbots is increasing. As health services become patient-centric, offering personalised and satisfactory experiences is of utmost priority for healthcare providers.
Many patients have questions and queries outside of typical business hours. AI can help provide around-the-clock support through chatbots that can answer basic questions and give patients resources when their provider’s office isn’t open. AI could also potentially be used to triage questions and flag information for further review, which could help alert providers to health changes that need additional attention.
As customer services take a whole new approach, providers are keen to invest in automated tools to offer timely care services. In the digital age, patients expect faster responses from healthcare service providers. E.g, Sensely: Conversational AI to improve health and drive member engagement which is a virtual nurse.
Integrating medical AI into clinician workflows can give providers valuable context while they're making care decisions. A trained machine learning algorithm can help cut down on research time by giving clinicians valuable search results with evidence-based insights about treatments and procedures while the patient is still in the room with them.
There are a lot of potential ways AI could reduce costs across the healthcare industry. Some of the most promising opportunities include reducing medication errors, customized virtual health assistance, fraud prevention, and supporting more efficient administrative and clinical workflows.
Payers and employers are using AI to help their members and employees access the benefits information they need and power robust analytics for performance insights.
With Watson Assistant for Health Benefits, health plans have a virtual assistant trained in conversational AI that can answer real-world questions about complex health plan benefits quickly and easily.
Treatment and Patient Outreach:
Designed to work with any EHR, IBM® Phytel® Outreach delivers automated, protocol-driven patient communications – helping providers efficiently identify and engage priority populations, drive annual wellness visits and close gaps in care.
Beyond scanning health records to help providers identify chronically ill individuals who may be at risk of an adverse episode, AI can help clinicians take a more comprehensive approach for disease management, better coordinate care plans and help patients to better manage and comply with their long-term treatment programmes.
Robots have been used in medicine for more than 30 years. They range from simple laboratory robots to highly complex surgical robots that can either aid a human surgeon or execute operations by themselves. In addition to surgery, they’re used in hospitals and labs for repetitive tasks, in rehabilitation, physical therapy and in support of those with long-term conditions.
DISEASE IDENTIFICATION & DIAGNOSTICS
“Early detection is the key for patient care”
AI is already being used to detect diseases Below are few examples
In Cancer detection : more accurately and in their early stages. According to the American Cancer Society, a high proportion of mammograms yield false results, leading to 1 in 2 healthy women being told they have cancer. The use of AI is enabling review and translation of mammograms 30 times faster with 99% accuracy, reducing the need for unnecessary biopsies
In detecting diabetic retinopathy
In Medical Imaging for Brain Tumours
New and improved techniques are being used in medical image registration to fasten the time taken to see growth in brain tumours. The details of the novel ML algorithm can be found at Faster analysis of medical images | MIT News | Massachusetts Institute of Technology
In Acute Kidney Injury(AKI)
Google’s DeepMind deep learning model for continuously predicting the future likelihood of a patient developing a life-threatening condition called acute kidney injury (AKI).
In Heart Failure
Unlike humans, AI never needs to sleep. Machine learning models could be used to observe the vital signs of patients receiving critical care and alert clinicians if certain risk factors increase. While medical devices like heart monitors can track vital signs, AI can collect the data from those devices and look for more complex conditions, such as sepsis
PERSONALIZED HEALTHCARE
Wearables and sensors are the enabling technology that provides data for AI and machine learning to personalise care.
Personalized medicine can provide for a more effective treatment based on the individual health data paired with predictive analytics. ML methods like supervised learning are used, which allows physicians to select from more limited sets of diagnoses and to estimate the given patient’s risk based on the similarity of symptoms and genetic information.
Perhaps the most valued quality of AI is its ability to dynamically learn and improve over time. As AI collects individual patient data, and begins to learn how patients react differently to feedback, it can begin tailoring feedback so that it’s personalised and predictive. Such feedback is the foundation upon which a preventive healthcare system is built.
Medtronic’s new IBM Watson-powered Sugar.IQ diabetes app uses real-time continuous glucose monitoring and insulin information from Medtronic pumps and glucose sensors to provide diabetes patients with personalised insights. The AI-based app is designed to learn from a patient’s own information input; its Glycemic Assist feature enables users to inquire about how specific foods or therapy-related actions and events impact their personal glucose levels. By following trends, Sugar.IQ can then help users discover the impact that these items have on their glucose levels. Patient inputs also enable Sugar.IQ to learn and issue blood glucose level predictions by assessing the patient’s current situation and the risk of glucose levels falling outside safe thresholds.
PREVENTIVE HEALTHCARE
“Prevention is better than cure.”
Clinical AI implementation promises a healthcare system that is preventive rather than reactionary. Today, patients are often diagnosed with a chronic condition, such as cancer or diabetes, when it’s too late to reverse the progression of the condition. Treatment plans with late stage diseases are expensive and debilitative. Patients are poorly equipped with feedback and insights into their own health conditions, and so are less proactive about making healthy lifestyle choices and adhering to physician advice. Hence, preventing the start of chronic disease and managing the disease post-diagnosis has become the focus of preemptive measures in healthcare.
A preventive healthcare system will capitalize on AI’s ability to collect, compile, and analyse data to facilitate three progressive and ultimately integrated stages of learning. First, AI will enable a broad scope of learning that will aid in more effective and efficient disease diagnostics based on historical data. The insights gained from this massive survey of Big Data will be utilised by physicians to further train AI. Second, AI will harness historical data and augment it with real-time patient data to provide feedback to patients. Finally, as AI begins to learn how patients react differently based on real-time data, it will create personalised and predictive feedback for each patient.
Medical-grade wearables with AI could create predictions based on a patient’s daily biometrics. If a heart disease patient is prone to developing a rapid heartbeat after X minutes of walking, then the medical device would make a prediction and alert the patient to avoid exceeding the recommended minutes of walking. AI could also exercise predictive capabilities by learning what kinds of feedback instigate adherence for a patient and then applying that feedback to improve the patient’s disease management, almost like a personal health coach. When patients can follow their own progress, and see how certain choices have a direct impact on their health, they are more likely to adhere to treatment plants, engage in their healthcare, and change their behaviour.
The following article is an interesting read.
PRECISION MEDICINE
“AI has the potential to provide customized real-time recommendations to patients around the clock.”
Precision medicine is an emerging approach for disease treatment and prevention that takes into account individual variability in genes, environment, and lifestyle for each person.
Precision Medicine is the broader, overall approach to developing treatments with better outcomes, based on clinical and molecular information (diagnostics), while Personalised Medicine is the patient-centered, treatment decision making clinical practices that result from this information. It is where doctors are able to utilize the information, and more targeted treatments, to improve treatment outcomes for their patients.
Precision medicine could become easier to support with virtual AI assistance. Because AI models can learn and retain preferences, AI has the potential to provide customized real-time recommendations to patients around the clock. Rather than having to repeat information with a new person each time, a healthcare system could offer patients around-the-clock access to an AI-powered virtual assistant that could answer questions based on the patient's medical history, preferences and personal needs.
TRAINING
AI allows those in training to go through naturalistic simulations in a way that simple computer-driven algorithms cannot. The advent of natural speech and the ability of an AI computer to draw instantly on a large database of scenarios, means the response to questions, decisions or advice from a trainee can challenge in a way that a human cannot. And the training programme can learn from previous responses from the trainee, meaning that the challenges can be continually adjusted to meet their learning needs.
And training can be done anywhere; with the power of AI embedded on a smartphone, quick catch up sessions, after a tricky case in a clinic or while travelling, will be possible.
Commentaires